About
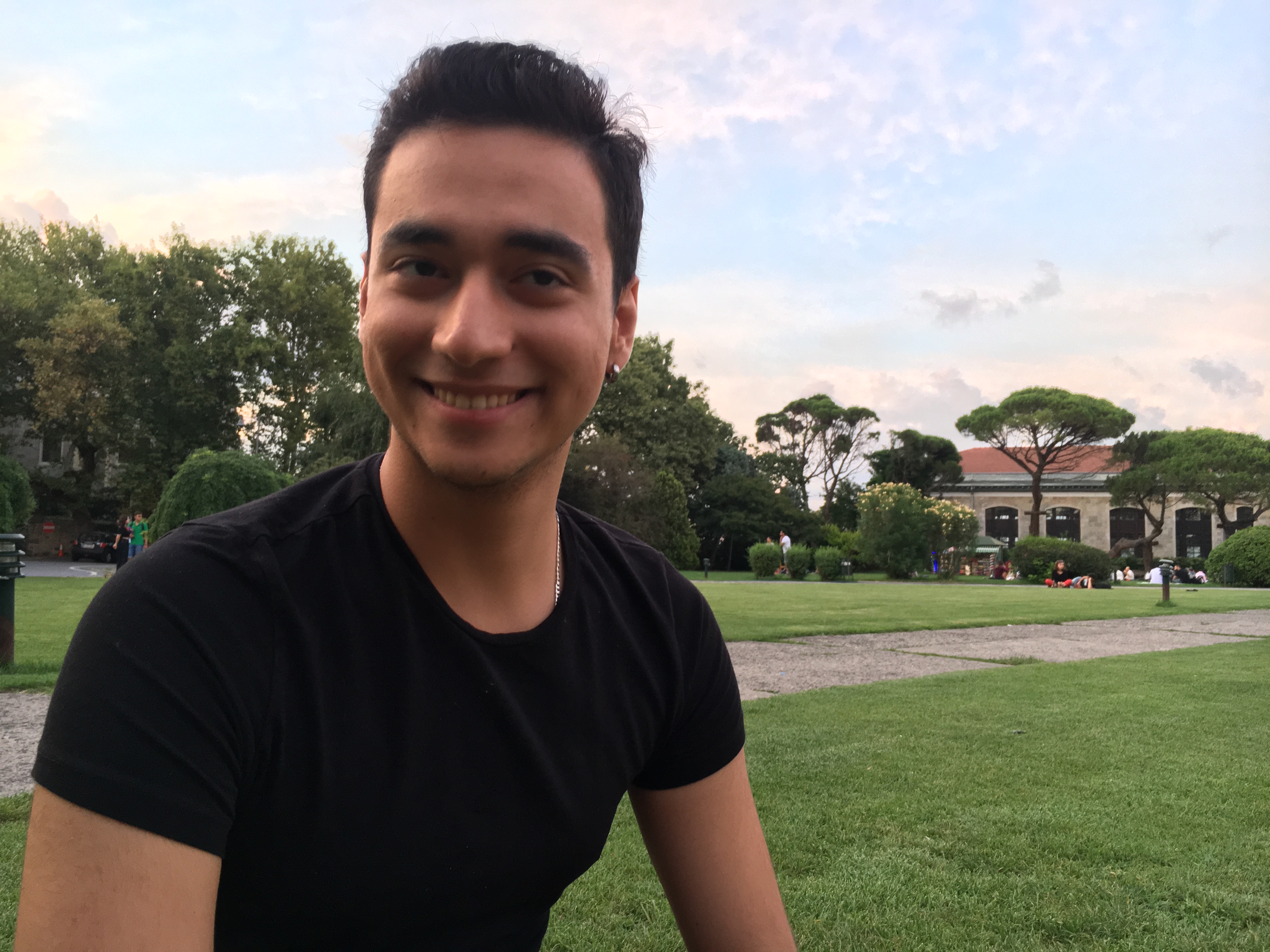
I am Gün Kaynar
I am Computer Science MSc student at Bilkent University where I am fortunate to work with Dr. Ercument Cicek. I am working as a Research and Teaching Assistant at CICEKLAB, Bilkent University, Ankara.
MSc in Computer Engineering at Bilkent University (2021-2024)
BSc in Molecular Biology and Genetics at Bogazici University (2015-2021)
Exchange student in Biology at Universitat de Barcelona (2020)
Izmir Atatürk High School (2011-2015)
My research interests include:
- Computational Biology
- Bioinformatics
- Biologically-Informed Models
- Deep Learning
- CNV Calling
- Cancer Biology
- NMR Spectroscopy
- Metabolomics
- Machine Learning
- Genomics
Publications
Journal Papers
ECOLE: Learning to call copy number variants on whole exome sequencing data
Nature Communications, 2024Mandiracioglu B, Ozden F, Kaynar G, Yilmaz MA, Alkan C, Cicek AE
Article, Scholar
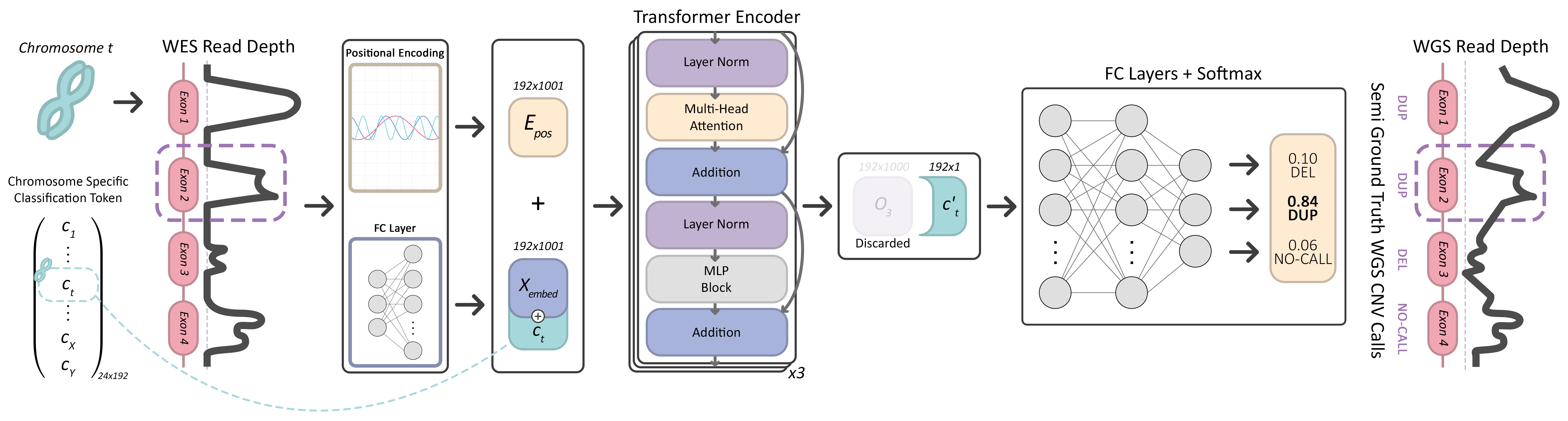
Abstract
Copy number variants (CNV) are shown to contribute to the etiology of several genetic disorders. Accurate detection of CNVs on whole exome sequencing (WES) data has been a long sought-after goal for use in clinics. This was not possible despite recent improvements in performance because algorithms mostly suffer from low precision and even lower recall on expert-curated gold standard call sets. Here, we present a deep learning-based somatic and germline CNV caller for WES data, named ECOLE. Based on a variant of the transformer architecture, the model learns to call CNVs per exon, using high-confidence calls made on matched WGS samples. We further train and fine-tune the model with a small set of expert calls via transfer learning. We show that ECOLE achieves high performance on human expert labeled data for the first time with 68.7% precision and 49.6% recall. This corresponds to precision and recall improvements of 18.7% and 30.8% over the next best-performing methods, respectively. We also show that the same fine-tuning strategy using tumor samples enables ECOLE to detect RT-qPCR validated variations in bladder cancer samples without the need for a control sample. ECOLE is available at https://github.com/ciceklab/ECOLE.
PiDeeL: Metabolic Pathway-Informed Deep Learning Model for Survival Analysis and Pathological Classification of Gliomas
Bioinformatics, 2023Kaynar G, Cakmakci D, Bund C, Todeschi J, Namer IJ, Cicek AE
Article, Scholar, ResearchGate
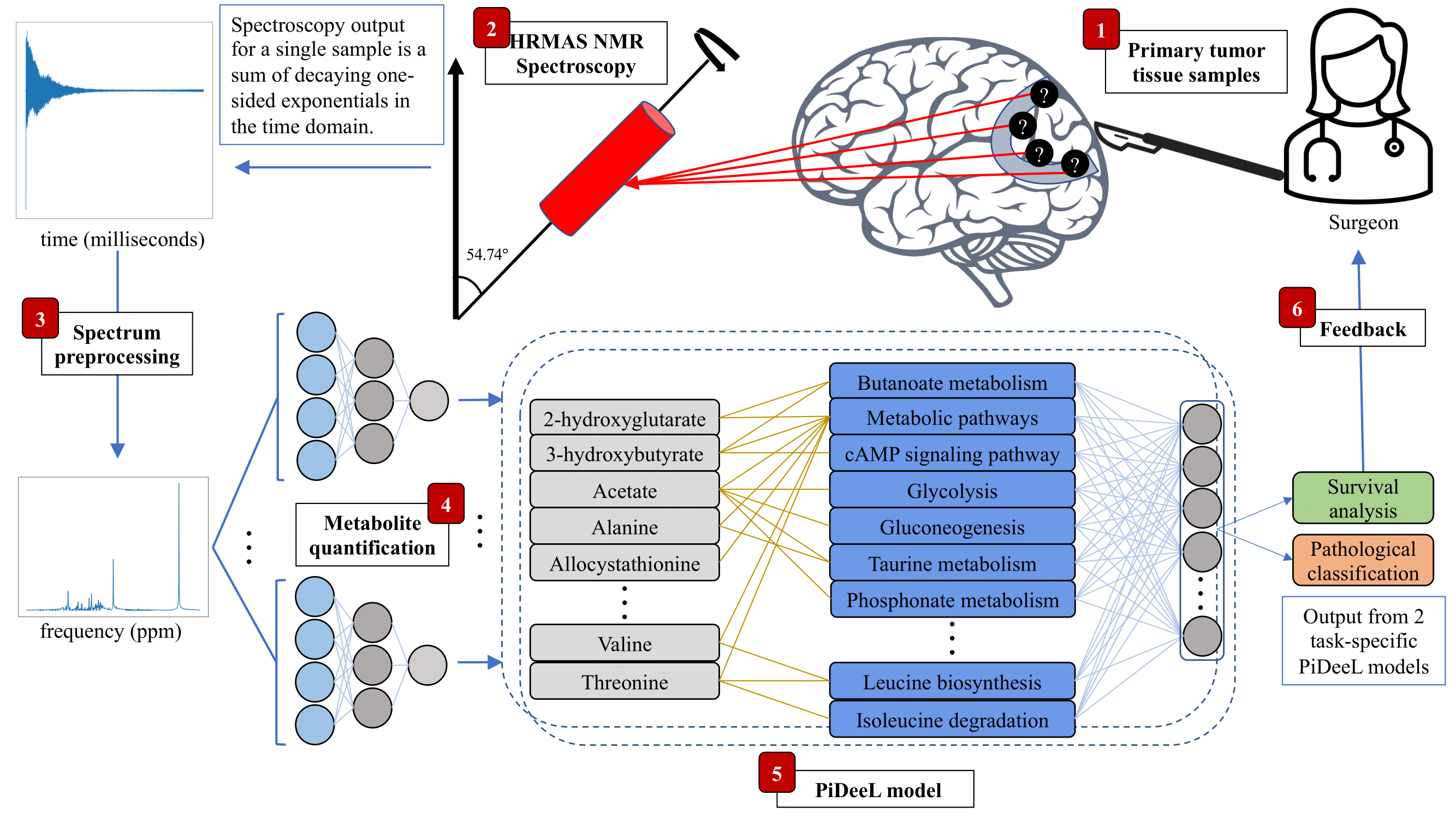
Abstract
Online assessment of tumor characteristics during surgery is important and has the potential to establish an intraoperative surgeon feedback mechanism. With the availability of such feedback, surgeons could decide to be more liberal or conservative regarding the resection of the tumor. While there are methods to perform metabolomics-based online tumor pathology prediction, their model complexity and, in turn, the predictive performance is limited by the small dataset sizes. Furthermore, the information conveyed by the feedback provided on the tumor tissue could be improved both in terms of content and accuracy. In this study, we propose a metabolic pathway-informed deep learning model, PiDeeL, to perform survival analysis and pathology assessment based on metabolite concentrations. We show that incorporating pathway information into the model architecture substantially reduces parameter complexity and achieves better survival analysis and pathological classification performance. With these design decisions, we show that PiDeeL improves tumor pathology prediction performance of the state-of-the-art in terms of the Area Under the ROC Curve (AUC-ROC) by 3.38% and the Area Under the Precision-Recall Curve (AUC-PR) by 4.06%. Similarly, with respect to the time-dependent concordance index (c-index), we observe that PiDeeL achieves better survival analysis performance (improvement up to 4.3%) when compared to the state-of-the-art. Moreover, we show that importance analyses performed on input metabolite features as well as pathway-specific hidden-layer neurons of PiDeeL provide insights into tumor metabolism. We foresee that the use of this model in the surgery room will help surgeons adjust the surgery plan on the fly and will result in better prognosis estimates tailored to surgical procedures. The code is released at https://github.com/ciceklab/PiDeeL. The data used in this study is released at https://zenodo.org/record/7228791.
Targeted Metabolomics Analyses for Brain Tumor Margin Assessment During Surgery
Bioinformatics, 2022Cakmakci D*, Kaynar G*, Bund C, Piotto M, Proust F, Namer IJ, Cicek AE
* = joint first authors
Article, Scholar, ResearchGate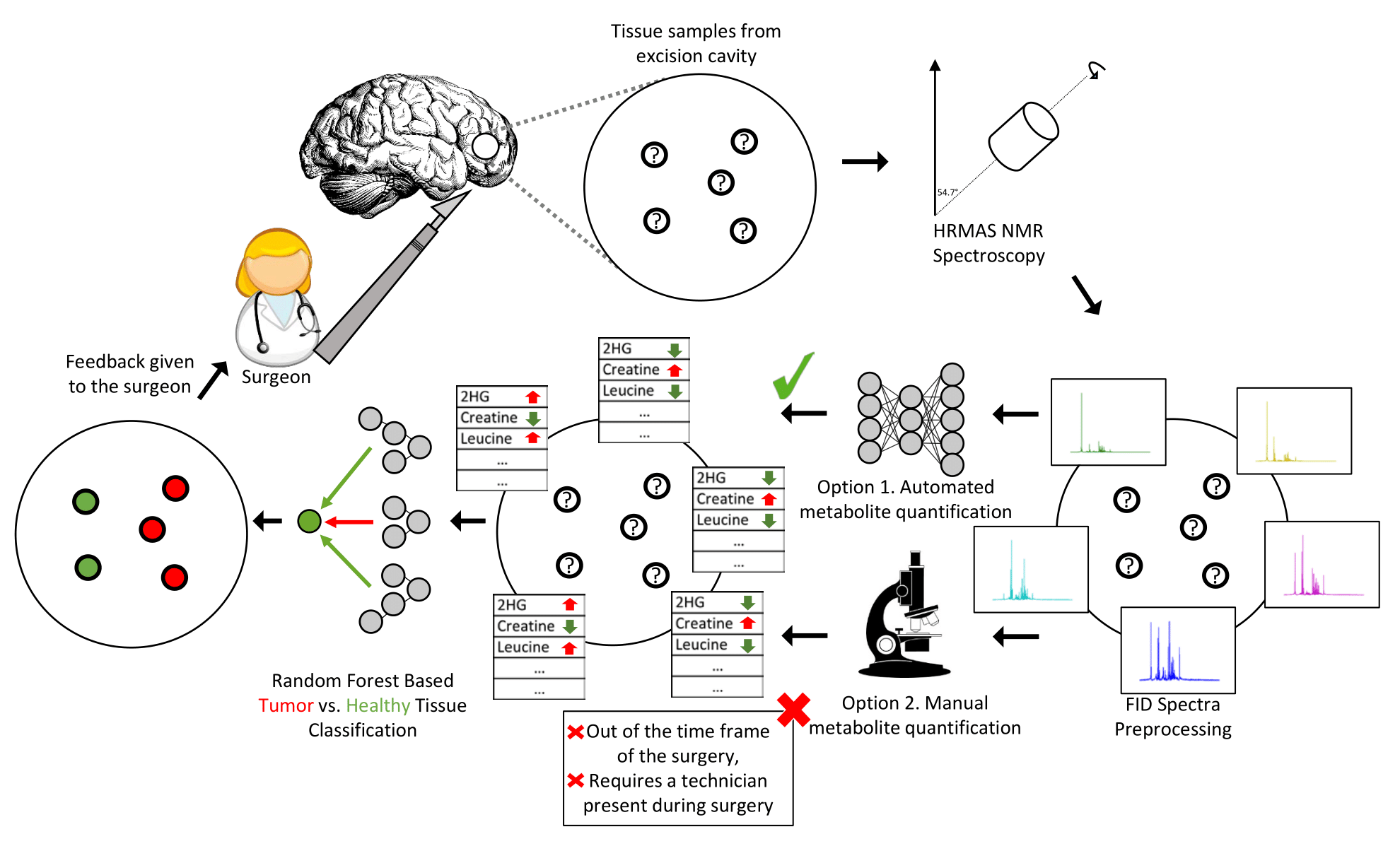
Abstract
Identification and removal of micro-scale residual tumor tissue during brain tumor surgery are key for survival in glioma patients. For this goal, High-Resolution Magic Angle Spinning Nuclear Magnetic Resonance (HRMAS NMR) spectroscopy-based assessment of tumor margins during surgery has been an effective method. However, the time required for metabolite quantification and the need for human experts such as a pathologist to be present during surgery are major bottlenecks of this technique. While machine learning techniques that analyze the NMR spectrum in an untargeted manner (i.e. using the full raw signal) have been shown to effectively automate this feedback mechanism, high dimensional and noisy structure of the NMR signal limits the attained performance. In this study, we show that identifying informative regions in the HRMAS NMR spectrum and using them for tumor margin assessment improves the prediction power. We use the spectra normalized with the ERETIC (electronic reference to access in vivo concentrations) method which uses an external reference signal to calibrate the HRMAS NMR spectrum. We train models to predict quantities of metabolites from annotated regions of this spectrum. Using these predictions for tumor margin assessment provides performance improvements up to 4.6% the Area Under the ROC Curve (AUC-ROC) and 2.8% the Area Under the Precision-Recall Curve (AUC-PR). We validate the importance of various tumor biomarkers and identify a novel region between 7.97 ppm and 8.09 ppm as a new candidate for a glioma biomarker. The code is released at https://github.com/ciceklab/targeted_brain_tumor_margin_assessment. The data underlying this article are available in Zenodo, at https://doi.org/10.5281/zenodo.5781769.
Peer-Reviewed Conference Proceedings
Poster, PiDeeL: Pathway-Informed Deep Learning Model for Survival Analysis and Pathological Classification of Gliomas
HIBIT (16th The International Symposium on Health Informatics and Bioinformatics), Ankara, Turkey, October 2023Kaynar G, Cakmakci D, Bund C, Todeschi J, Namer IJ, Cicek AE
Poster, bioRxiv, Scholar, ResearchGate
Conference Paper, ECOLE: Learning to call copy number variants on whole exome sequencing data
HIBIT (16th The International Symposium on Health Informatics and Bioinformatics), Ankara, Turkey, October 2023Mandiracioglu B, Ozden F, Kaynar G, Yilmaz MA, Alkan C, Cicek AE
bioRxiv, Scholar
Conference Paper, Pathway-informed deep learning model for survival analysis and pathological classification of gliomas
RECOMB-CCB (The 15th RECOMB Satellite Workshop on Computational Cancer Biology), Istanbul, Turkey, April 2023.Kaynar G, Cakmakci D, Bund C, Todeschi J, Namer IJ, Cicek AE
Talk, bioRxiv, Scholar, ResearchGate
Poster, Targeted Metabolomics Analyses for Brain Tumor Margin Assessment During Surgery
RECOMB (27th Annual International Conference on Research in Computational Molecular Biology), Istanbul, Turkey, April 2023.Cakmakci D, Kaynar G, Bund C, Piotto M, Proust F, Namer IJ, Cicek AE
Poster, Article, Scholar, ResearchGate
Academic
Experience
Computer Engineering Department, CICEKLAB, Bilkent University, Ankara, Turkey
Computer Engineering Department, Bilkent University, Ankara, Turkey
CICEKLAB, under the supervision of Dr. Ercument Cicek,
Computer Engineering Department, Bilkent University, Ankara, Turkey
Center for Proteomics and Bioinformatics, under the supervision of Prof. Mark Chance,
Case Western Reserve University, Cleveland, OH, USA.
Reviewer
Volunteer
Teaching
Software and
Paper Implementations
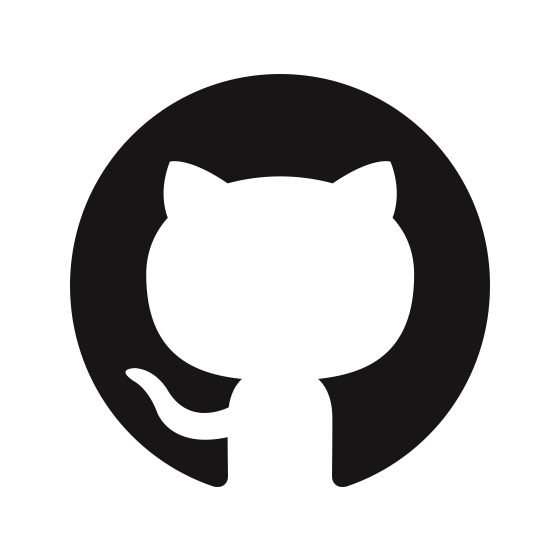
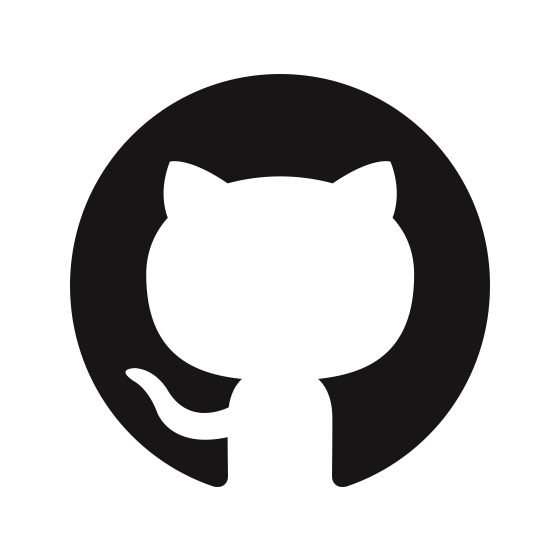
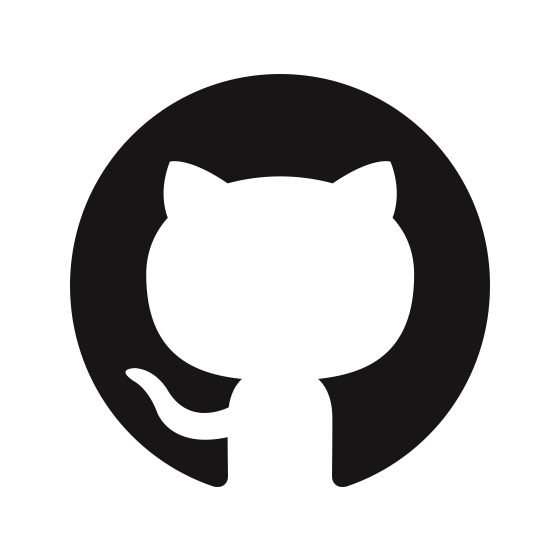
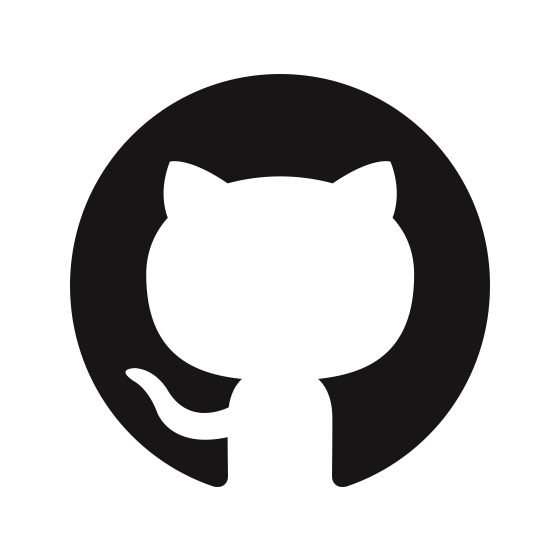
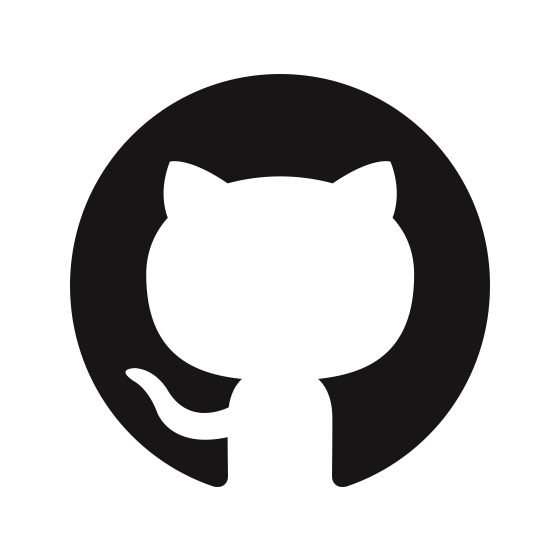